Alessandra Corsi and a group of high-profile researchers are working to see how artificial intelligence can solve an information-sharing challenge.
Alessandra Corsi never met a scientific challenge she wouldn’t tackle.
In that spirit, Corsi, a professor in the Department of Physics and Astronomy at Texas Tech University, has taken on a collaborative research project that focuses on data science but with application to her research bailiwick of multi-messenger astronomy.
Corsi is part of a team that recently received a $10 million grant from the U.S. Department of Energy. The five-year project is aimed at employing artificial intelligence (AI) to protect and share centralized data without violating proprietary standards that typically safeguard such sensitive information.
The group has only just begun its work and a future meeting of the collaborators could take place on the Texas Tech campus in the spring.
The hope is to use AI to generate a method of sharing data among researchers without centralizing or compromising it. AI could be used to not only house information but also “learn” from the data. AI would do the learning, and the only thing shared would be the physics involved.
“This brings me a bit out of my standard research field,” Corsi said. “The interest is in partnering with people doing more AI-oriented science, and I was approached by a colleague at the Argonne National Laboratory who is involved in research related to making systems more resilient to failure. I am very interested in AI and exploring this.”
Corsi is a co-principal investigator for the project that includes researchers from Argonne, a federally funded laboratory in Illinois, the Oak Ridge National Laboratory in Tennessee and the SLAC National Accelerator Laboratory in California. The group’s focus will be on taking resources such as remote computers and data stores and developing uninterrupted processing of data streams.
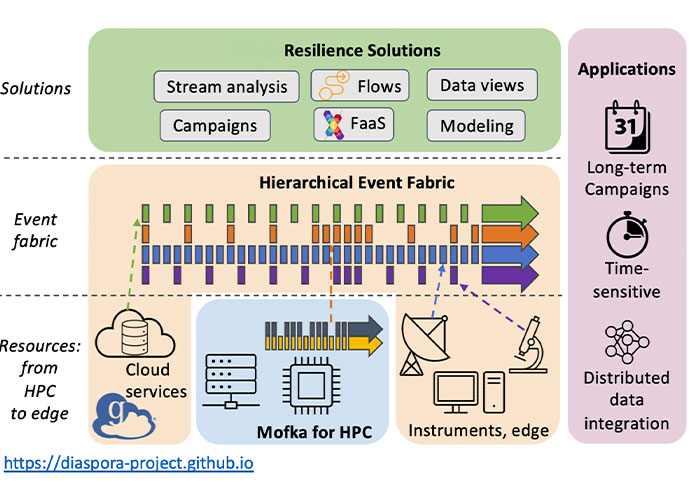
The primary concern is systems can sometimes fail without notice, placing an entire project in jeopardy and making resiliency and instant communication priorities.
“We are looking at distributed computing or distributed experiments taking place on different computers,” she said. “The question is how do you make things efficient so if one of your resources fails, you know how to redistribute the task so the whole thing doesn’t fail? The problem is a local failure might result in a local message and a whole process might die without you knowing it.”
The project is the perfect vehicle for AI, an expanding and relatively new technology that has opened opportunities in numerous fields, including data science. Beyond that, though, Corsi sees ways to integrate what is learned into multi-messenger astronomy. In this field, data is often collected across various telescopes and instruments operating on different wavelengths each with their own proprietary rights.
The challenge for the astrophysicist is pulling the information together and then interpreting it based on the physics behind a cosmic event like an explosion causing a gravitational wave.
“NASA might deliver the data right away, but the VLA (Very Large Array, a collection of huge telescopes in New Mexico) might have a one-year limit of proprietary time,” she said. “The information is not public and is submitted and collected in different ways. Something has exploded, but you have only partial data so how do you bring all the information together so as not to lose the opportunity to learn?”
The hope is to possibly use AI to generate a method of sharing data among researchers without centralizing or compromising it. AI could be used to not only house information but also “learn” from the data. AI would do the learning, and the only thing shared would be the physics involved.
“It is an ambitious goal that we have,” she said. “We have AI models that can sift through gravitational wave data as far as what some of our collaborators have worked on. But it is only baby steps we are taking right now.”
For now, researchers are providing AI models with existing findings to see how quickly they can apply what is learned. At some point, AI could be used to pinpoint optimal times for researchers to use telescope time, letting them know when new observations could be most useful to extract information about an astrophysical event of interest.
Data science is a rapidly growing area with applications across numerous other fields, including physics and astronomy. Simply put, data science involves exploration of huge data sets with statistical tools, making it an increasingly attractive field of study inside and outside of academia.
“It has broad application, which is why data science is so powerful,” Corsi said. “You could learn data science or AI techniques in the context of astronomy, but you don’t have to stay attached to that because whatever you learn might apply to any big data problem.”
The blending of data science, physics and astronomy makes the project attractive on several fronts, including an appeal to students looking for one more path into science.
“This project is a chance to enlarge the population of people who are into multi-messenger astronomy,” she said. “Practically, we strive to bring research into our classes early but that is limited to our astronomy classes right now, so in the future maybe we offer data science classes with a flavor of astronomy.
“That would give us tools to show real application from this project that could become an in-class activity. We have talked about a data analysis course in Physics and Astronomy that could give students a flavor of all that is covered and what’s common among different fields.”